Maxime Labonne PRO
AI & ML interests
Recent Activity
Articles
Organizations
mlabonne's activity
that looks great, well done!
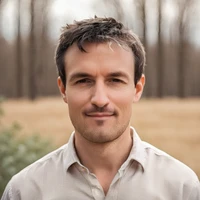
Hi all, I created this "AutoGen Multi-Agent Workflow" space that allows you to experiment with multi-agent workflows.
By default, it allows code generation with built-in quality control and automatic documentation generation. It achieves this by leveraging multiple AI agents working together to produce high-quality code snippets, ensuring they meet the specified requirements.
In addition to the default, the space allows users to set custom system messages for each assistant, potentially completely changing the workflow.
# Workflow Steps
1. User Input:
- The user defines a prompt, such as "Write a random password generator using python."
- Outcome: A clear task for the primary assistant to accomplish.
2. Primary Assistant Work:
- The primary assistant begins working on the provided prompt.
It generates an initial code snippet based on the user's request.
- Outcome: An initial proposal for the requested code.
3. Critic Feedback:
- The critic reviews the generated code provides feedback or (if the output meets the criteria), broadcasts the APPROVED message.
(This process repeats until the output is APPROVED or 10 messages have been exchanged).
- Outcome: A revised Python function that incorporates the critic's feedback.
4. Documentation Generation:
- Once the code is approved, it is passed to a documentation assistant.
The documentation assistant generates a concise documentation for the final code.
- Outcome: A short documentation including function description, parameters, and return values.
Enjoy!
CultriX/AutoGen-MultiAgent-Example
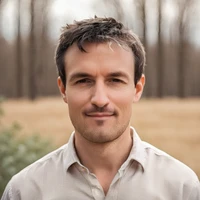
👷 It focuses on practical use cases, so if you’re working on something, bring it along.
👯♀️ It’s peer reviewed and open so you can discuss and get feedback.
🤘 If you’re already a smol pro, feel free to drop a star or issue.
> > Part 1 starts now, and it’s on instruction tuning!
https://github.com/huggingface/smol-course
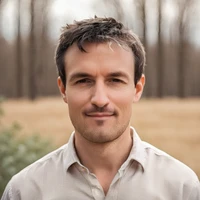
In this post, I replicate the process @mlabonne used to clean the new microsoft/orca-agentinstruct-1M-v1 dataset.
The cleaning process consists of:
- Joining the separate splits together / add split column
- Converting string messages into list of structs
- Removing empty system prompts
https://huggingface.co/blog/cfahlgren1/the-beginners-guide-to-cleaning-a-dataset
Here's his new cleaned dataset: mlabonne/orca-agentinstruct-1M-v1-cleaned
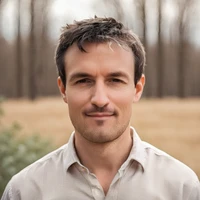
1. We integrate Post-Training Static Quantization using OpenVINO, a very efficient solution for CPUs that processes 4.78x as many texts per second on average, while only hurting performance by 0.36% on average. There's a new
export_static_quantized_openvino_model
method to quantize a model.2. We add the option to train with prompts, e.g. strings like "query: ", "search_document: " or "Represent this sentence for searching relevant passages: ". It's as simple as using the
prompts
argument in SentenceTransformerTrainingArguments
. Our experiments show that you can easily reach 0.66% to 0.90% relative performance improvement on NDCG@10 at no extra cost by adding "query: " before each training query and "document: " before each training answer.3. Sentence Transformers now supports training PEFT adapters via 7 new methods for adding new adapters or loading pre-trained ones. You can also directly load a trained adapter with SentenceTransformer as if it's a normal model. Very useful for e.g. 1) training multiple adapters on 1 base model, 2) training bigger models than otherwise possible, or 3) cheaply hosting multiple models by switching multiple adapters on 1 base model.
4. We added easy evaluation on NanoBEIR, a subset of BEIR a.k.a. the MTEB Retrieval benchmark. It contains 13 datasets with 50 queries and up to 10k documents each. Evaluation is fast, and can easily be done during training to track your model's performance on general-purpose information retrieval tasks.
Additionally, we also deprecate Python 3.8, add better compatibility with Transformers v4.46.0, and more. Read the full release notes here: https://github.com/UKPLab/sentence-transformers/releases/tag/v3.3.0
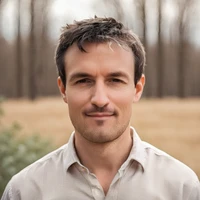
Haha thanks for this suggestion @tachyphylaxis but @failspy is the one who coined the name "abliteration". He has full responsibility for the chaos he unleashed, I'm barely a messenger here.
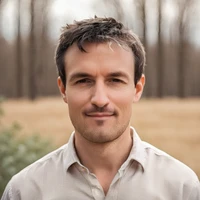
1️⃣ ONNX Backend: This backend uses the ONNX Runtime to accelerate model inference on both CPU and GPU, reaching up to 1.4x-3x speedup depending on the precision. We also introduce 2 helper methods for optimizing and quantizing models for (much) faster inference.
2️⃣ OpenVINO Backend: This backend uses Intel their OpenVINO instead, outperforming ONNX in some situations on CPU.
Usage is as simple as
SentenceTransformer("all-MiniLM-L6-v2", backend="onnx")
. Does your model not have an ONNX or OpenVINO file yet? No worries - it'll be autoexported for you. Thank me later 😉🔒 Another major new feature is Static Embeddings: think word embeddings like GLoVe and word2vec, but modernized. Static Embeddings are bags of token embeddings that are summed together to create text embeddings, allowing for lightning-fast embeddings that don't require any neural networks. They're initialized in one of 2 ways:
1️⃣ via Model2Vec, a new technique for distilling any Sentence Transformer models into static embeddings. Either via a pre-distilled model with
from_model2vec
or with from_distillation
where you do the distillation yourself. It'll only take 5 seconds on GPU & 2 minutes on CPU, no dataset needed.2️⃣ Random initialization. This requires finetuning, but finetuning is extremely quick (e.g. I trained with 3 million pairs in 7 minutes). My final model was 6.6% worse than bge-base-en-v1.5, but 500x faster on CPU.
Full release notes: https://github.com/UKPLab/sentence-transformers/releases/tag/v3.2.0
Documentation on Speeding up Inference: https://sbert.net/docs/sentence_transformer/usage/efficiency.html
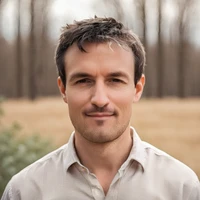
I first learned about instruction-based classifiers like BERT-NLI 3-4 years ago, through the @HuggingFace ZeroShotClassificationPipeline. Digging deeper into this, it was surprisingly easy to find new datasets, newer base models, and reusable fine-tuning scripts on the HF Hub to create my own zeroshot models - although I didn't know much about fine-tuning at the time.
Thanks to the community effect of the Hub, my models were downloaded hundreds of thousands of times after a few months. Seeing my research being useful for people motivated me to improve and upload newer models. Leaving my contact details in the model cards led to academic cooperation and consulting contracts (and eventually my job at HF).
That's the power of open science & open source: learning, sharing, improving, collaborating.
I mean every word in my thesis acknowledgments (screenshot). I'm very grateful to my supervisors @vanatteveldt @CasAndreu @KasperWelbers for their guidance; to @profAndreaRenda and @CEPS_thinktank for enabling me to work part-time during the first year; to @huggingface for creating awesome tools and an awesome platform; and to many others who are not active on social media.
Links to the full thesis and the collection of my most recent models are below.
PS: If someone happens to speak Latin, let me know if my diploma contains some hidden Illuminati code or something :D
Thanks @Tonic ! Sorry, there's no other way to access the API at the moment :( Hopefully, it's just temporary
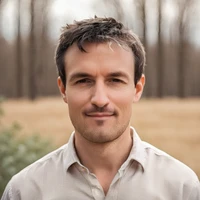
Thanks a lot @Tonic !
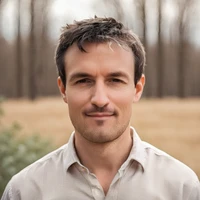
testing it out now to make a dataset , i cant hardly wait... but one question 👇🏻 why / wen ? 😅🚀🚀
check out the blog post : https://www.liquid.ai/liquid-foundation-models
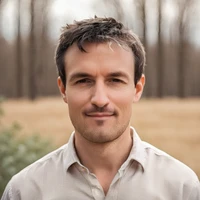
I modified it, thanks again. I recommend using the original model for strong instruction-following capabilities. Self-merges tend to suffer, especially around skills related to reasoning.
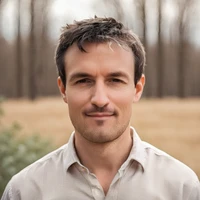
Thanks a lot, I've added your feedback to the model card: https://huggingface.co/mlabonne/BigQwen2.5-125B-Instruct
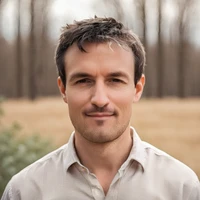
I haven't. That's nice, thanks for your feedback. Do you mind sharing the prompt and answer if possible? I'd like to understand what it's good at.
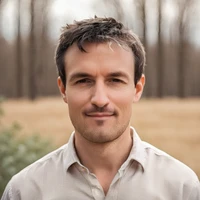
Hey @kweel , thanks for your message. First, I want to say that "abliteration" can be used in many, many ways, and uncensoring models is just one of them (see @failspy 's https://huggingface.co/failspy/Llama-3-8B-Instruct-MopeyMule).
I agree that "disabling refusals" and "uncensoring" are not the same thing, but disabling refusals is kind of a superset of uncensoring here. To me, the limitations are more connected to the single direction we target, the lack of high-quality calibration sets, and the performance drop it creates.
Nice work, congrats!
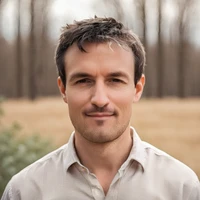
Lately, I've spent some time fine-tuning language models.
Now I am happy to release Phi 3.5 mini ITA: a fine-tuned version of Phi-3.5-mini-instruct to improve performance on the Italian language
🔹 Small (3.82 B parameters) but capable model
🔹 128k context length
Chat with it on 🤗 Spaces: anakin87/Phi-3.5-mini-ITA
Model card: anakin87/Phi-3.5-mini-ITA
🗃️ Data
Supervised fine-tuning using a good mix of English and Italian data:
- mlabonne/FineTome-100k by @mlabonne
- efederici/capybara-claude-15k-ita by @efederici
🙏 Thanks to the authors for the datasets.
🎯 Targeted training with Spectrum
I used Spectrum, a relatively new technique for parameter-efficient learning.
The idea is to train only the layers of the model with high Signal-to-Noise Ratio (SNR) and ❄️ freeze the rest.
I trained the top 30% of model layers.
📝 Spectrum paper: https://arxiv.org/abs/2406.06623
📊 Vibe check and performance on Italian benchmarks seem encouraging
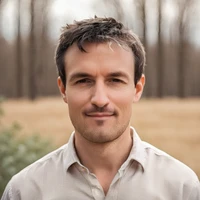
That's an interesting project. The abliteration process relies on the assumption that refusal in LLMs is mediated by a single direction. I don't expect the concept of "cat" to be as simple, however. You could maybe try to narrow your scope?
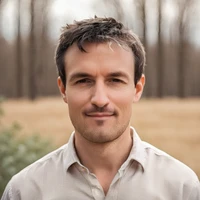
Today I decided to see if that matters, and the results have me.. for lack of a better word, perplexed
My setup:
Mistral Nemo Instruct 2407
- convert to FP32, calculate imatrix, quantize to Q8_0 and Q4_K_M
- convert to FP16, calculate imatrix, quantize to Q8_0 and Q4_K_M
I calculated the kld base from the FP32 model:
./llama-perplexity -m /models/Mistral-Nemo-Instruct-2407-f32.gguf -f /training_data/wikitext-2-raw/wiki.test.raw --kl-divergence-base /training_data/mistral-nemo-f32.kld -ngl 35 -fa -sm row
then calculated the divergence itself for each like so:
./llama-perplexity -m /models/Mistral-Nemo-Instruct-2407-Q8_0.gguf -f /training_data/wikitext-2-raw/wiki.test.raw --kl-divergence-base /training_data/mistral-nemo-f32.kld --kl-divergence -ngl 50 -fa -sm row
Q4_K_M from fp16 and fp32 were similar, trading blows across statistics, odd since i expected fp32 to be strictly better but it's not
Q8_0 is where things get weird. Despite each file being slightly different size, and the sha256sum of course being different, they each get *completely identical* scores, down to 6 decimal places of precision on the statistics.
How is this possible? Is there something I don't understand about llama.cpp that makes it always convert to fp16 before it does quantization? Am I wasting time using FP32/BF16??