Stefan Schweter PRO
stefan-it
AI & ML interests
Flair Library, NER & PoS Tagging, LM Pretraining (mostly encoder-only), Historical Language Models
Recent Activity
reacted
to
davanstrien's
post
with 🚀
1 day ago
The https://huggingface.co/datasets/data-is-better-together/fineweb-c dataset is growing!
This week a few more languages have got 1,000 annotations for the educational quality of data from https://huggingface.co/datasets/HuggingFaceFW/fineweb-2.
Why should you care?
The quality of pre-training data can have a big impact on the performance of downstream language models trained on that data (https://huggingface.co/spaces/HuggingFaceFW/blogpost-fineweb-v1).
Being able to filter by educational quality is on way of improving the quality of the data you use for training an LLM. Very importantly this approach can also reduce the amount of data needed for pertaining.
Why not use an LLM?
LLMs can be used to annotate educational quality for a subset of data. This data can then be used to train a smaller encoder only model to label the full dataset. However, this may not work well for languages outside of english. This is where fineweb-c (community) comes in.
The community is annotating the educational quality of fineweb2 data. Currently 114 languages have some annotations. These annotations will enable a number of things:
- Evaluate whether an LLM can label the educational quality for texts in that language well
- Directly be used for training quality classifiers
- Help discover other rules and huerisitcs for refining fineweb2 further for different languages.
This week the following languages where done:
Swedish thanks to: @Lauler @AntonVic @ohallstrom @bjarlestam @menbom @Ekgren @apsod
Ukrainian thanks to: @hannayukhymenko @robinhad @realPivo @RabotiahovDmytro @reciprocate
Assamese thanks to: @moyoor97 @Arpanjyoti @nawaf-helmi123 @pahigogoi1 @aelhence @kishorekashyap
Want to learn more: https://huggingface.co/blog/davanstrien/fineweb2-community
Contribute yourself here: https://huggingface.co/spaces/data-is-better-together/fineweb-c
commented
a paper
1 day ago
Building Foundations for Natural Language Processing of Historical
Turkish: Resources and Models
Articles
Organizations
Posts
1
Post
1214
My latest project is the outcome of the last 2+ years working with TPUs from the amazing TPU Research Cloud (TRC) program and training Encoder-only LMs with the TensorFlow Model Garden library.
👉 Link: https://github.com/stefan-it/model-garden-lms
An overview of some features:
- Cheatsheet for setting-up a TPU VM Pod (with all necessary dependencies) to pretrain LMs with TF Model Garden
- Conversion scripts that convert TF Model Garden weights to Hugging Face Transformers-compatible models
- Supported architectures include BERT, BERT with Token Dropping and TEAMS
I also released BERT-based models pretrained on the great Hugging Face FineWeb and FineWeb-Edu datasets (10BT subset). With more to come!
👉 Model Hub Link: https://huggingface.co/model-garden-lms
If you find these resources useful, please give them a like!
Made from Bavarian Oberland with ❤️ and 🥨.
👉 Link: https://github.com/stefan-it/model-garden-lms
An overview of some features:
- Cheatsheet for setting-up a TPU VM Pod (with all necessary dependencies) to pretrain LMs with TF Model Garden
- Conversion scripts that convert TF Model Garden weights to Hugging Face Transformers-compatible models
- Supported architectures include BERT, BERT with Token Dropping and TEAMS
I also released BERT-based models pretrained on the great Hugging Face FineWeb and FineWeb-Edu datasets (10BT subset). With more to come!
👉 Model Hub Link: https://huggingface.co/model-garden-lms
If you find these resources useful, please give them a like!
Made from Bavarian Oberland with ❤️ and 🥨.
Collections
14
My pretrained LMs on FineWeb datasets - part of my TensorFlow Model Garden LMs project
A Collection of Historical Multilingual Language Models
-
dbmdz/bert-base-historic-multilingual-cased
Fill-Mask • Updated • 30 • 6 -
dbmdz/bert-base-historic-multilingual-64k-td-cased
Fill-Mask • Updated • 18 • 1 -
hmbyt5-preliminary/byt5-small-historic-multilingual-span20-flax
Text2Text Generation • Updated • 58 -
hmteams/teams-base-historic-multilingual-discriminator
Updated • 3
models
1334
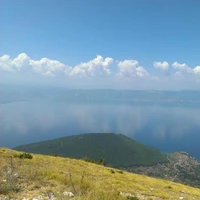
stefan-it/bert5urk
Updated
•
5
•
2
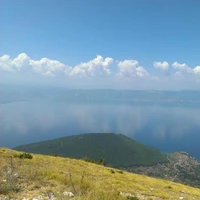
stefan-it/span-marker-gelectra-large-germeval14
Token Classification
•
Updated
•
345
•
2
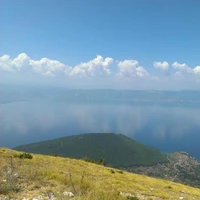
stefan-it/zeitungs-lm-v1
Updated
•
24
•
3
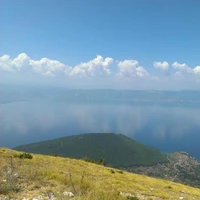
stefan-it/wav2vec2-large-xlsr-53-basque
Automatic Speech Recognition
•
Updated
•
717
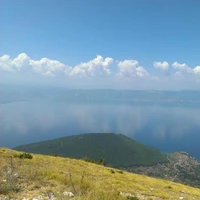
stefan-it/german-gpt2-larger
Text Generation
•
Updated
•
423
•
8
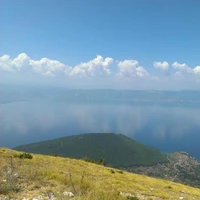
stefan-it/xlstm-german-wikipedia
Text Generation
•
Updated
•
47
•
7
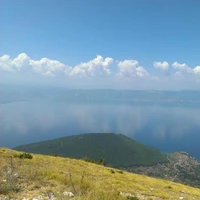
stefan-it/flair-barner-wiki-coarse-gbert-large
Token Classification
•
Updated
•
7
•
1
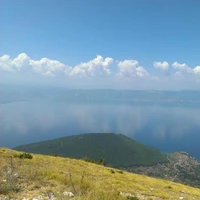
stefan-it/flair-clean-conll-5
Token Classification
•
Updated
•
6
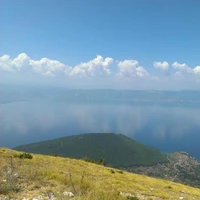
stefan-it/flair-clean-conll-4
Token Classification
•
Updated
•
2
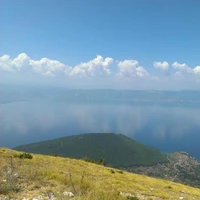
stefan-it/flair-clean-conll-3
Token Classification
•
Updated
datasets
12
stefan-it/senti-anno
Viewer
•
Updated
•
929
•
112
stefan-it/offenseval2020_tr
Viewer
•
Updated
•
35.3k
•
140
stefan-it/dewiki-20230701-nltk-corpus
Viewer
•
Updated
•
39.4M
•
44
•
2
stefan-it/germeval14_no_wikipedia
Preview
•
Updated
•
51
stefan-it/histnero
Viewer
•
Updated
•
217k
•
35
stefan-it/HisGermaNER
Preview
•
Updated
•
183
•
2
stefan-it/co-funer
Preview
•
Updated
•
38
stefan-it/german-dbmdz-bert-corpus
Viewer
•
Updated
•
52.8M
•
71
•
2
stefan-it/span-marker-base-model-detection
Viewer
•
Updated
•
28
•
37
stefan-it/flair-base-model-detection
Viewer
•
Updated
•
52
•
32
•
1